Webinar Recap: Applied AI for Enhanced Clinical Data Operations
Subscribe
Get our content delivered to your inbox.
Subscribe
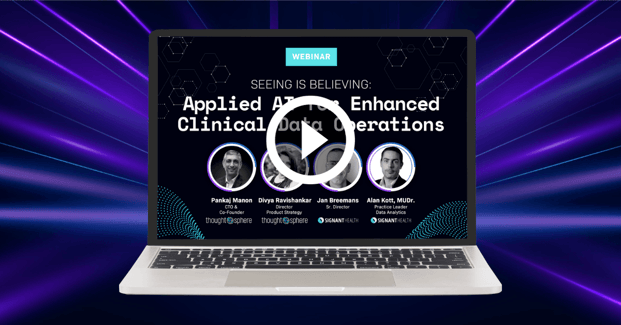
If you missed Signant’s September 20 webinar on artificial intelligence (AI) use cases and practical applications for clinical trial data management, it’s not too late. You can watch the recorded webinar here.
We’ve also summarized the key takeaways and expert insights for you.
Webinar Takeaways
Our speakers agreed AI can provide a solid foundation for data aggregation, which can be helpful in several stages of a study. There were many additional webinar takeaways, including:
- AI enables more efficient and accurate data aggregation and harmonization, accelerating study startup and reviews while offering more opportunities to data-driven decision-making.
- AI algorithms can learn from configurations, or rules, humans set in regard to data processing, and will automatically make adjustments for the subsequent execution.
- There are unsupervised learning models that identify multivariate outliers as a way to identify data discrepancies.
- Customers who’ve utilized AI for data ingestion, instead of the traditional warehouse approach, saw significant improvements in data quality and it took less time to deploy so they can review the data sooner than usual.
- AI can use current, historic, and real-word data for creating hypotheses, performing tests, or setting benchmarks.
- When you’re equipped with the actual and projected count information, you can proactively work on reducing the risk of a possible threshold, and you can implement corrective action.
- The application of AI is especially useful in early-phase trials, certain psychiatric trials, and trials with schedule one and two drugs in which there is a higher likelihood for the same person to enroll in the study multiple times.
- AI can help us shuffle through the data that is being collected and make a machine verification of the suitability of the subject. It can help us flag for additional reviews, subjects who may be questionable and then help sponsor decide whether this such a subject should participate in the trial or potentially not.
Meet the experts
Jan Breemans, Signant’s Senior Director of Global Solutions, kicked things off by discussing how the industry has been talking about the benefits of AI and machine learning for a while. However, he noted, we haven’t always seen examples that bring practical value in settings that fit our needs. As a result, AI could be seen as a buzzword, instead of a legitimate solution, which is why we wanted to bring experts to present you with practical AI use cases.
Pankaj Manon, Thoughtsphere Chief Technology Officer, explained how AI is applied to the clinical data chain. Beginning with ingestion from many data sources to aggregation, harmonization, and standardization, AI automates and streamlines these processes while simultaneously applying validation and conformance checks aligned with study design. The AI model also learns based on how humans update the configurations, adjusting subsequent outputs. AI-driven data ingestion, mapping, and validation results in faster startups and reviews.
Divya Ravishankar, Director of Product Strategy for Thoughtsphere, shared an example where machine learning was applied for monitoring of quality tolerance limits. In this case, the algorithm generated a projection line contrasted against the actual and expected for that study. So, the users saw a holistic picture and could take preventative measures when needed.
Alan Kott, MUDr., Signant’s Practice Leader for Data Analytics, pointed out that with machine learning, we have, for the first time, the opportunity not only to identify data quality concerns as we have been doing for years now using traditional analytical methods, but we also now have the ability to predict future data quality concerns and address them before they propagate throughout a study.